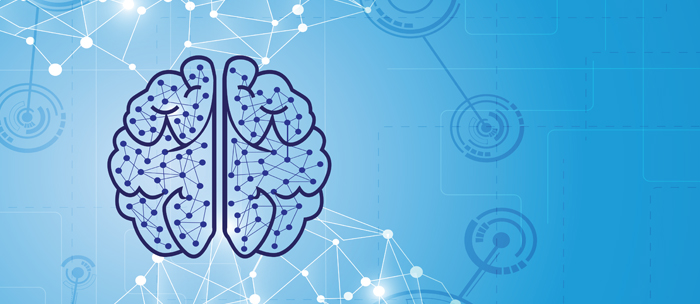
AI. It’s one of the hottest topics in advertising these days. It seems like every ad tech firm is promoting their AI acumen.
As with “big data” a year or so ago though, it can be hard to separate the truth from bluster. Some argue that there isn’t enough data to make AI effective for advertising and AI is merely the industry’s latest buzzword.
To get a different perspective on this issue, we talked to two professors who specialize in AI, Richard Baraniuk of Rice University and Tim Kraska of Brown University. We interviewed both separately. Excerpts of those conversations appear below:
MM: What is the difference between deep learning and machine learning?
Richard Baraniuk: Deep learning is just a special case or an example of a certain kind of machine learning. Machine learning is a broad classification of a set of techniques for solving certain kinds of problems that involve using data to construct systems that can aid in solving problems like making decisions. Deep learning is just a particular example of one machine learning technique.
Tim Kraska: Deep learning is a sub-field of machine learning. It’s one very particular type. Machine learning is a bunch of techniques, including regression models. They are different types of algorithms you can use. The most traditional ones would be linear regressions, polynomial regressions. They are ways to find data to explain your model to predict future events while deep learning is a very different type of model building which inspired by what the brain does.
MM: How would deep learning come up with different solutions than machine learning?
RB: Let me think of a good analogy…Machine learning is like talking about automobiles and deep learning would be talking about Ford, which is a particular kind of automobile. So a particularly powerful set of algorithms in machine learning are these deep learning methods. It’s basically a rebranding of neural networks or artificial neural networks which folks have been working on for 60 years or more and are very loosely based on how the brain works or might work. The reason they’re called deep learning algorithms is you take some basic machine learning algorithm and you connect it to another one and connect it to another one and so forth so you make this cascade or chain of many algorithms in a row and that creates one of these so-called deep learning approaches. And they are probably the hype-iest of machine learning approaches today.
MM: Facebook’s News Feed is often cited as an example of machine learning. Is that correct?
RB: Exactly. Any mathematical algorithm that’s able to use data and learn from that data in order to do an even better job next time, that’s a machine learning technique.
TK: Machine learning nowadays is in almost every type of product you can think of. If you go to Netflix and get a video recommendation, that’s machine learning. If you go to a store on your phone and it makes a recommendation of what to buy next, that’s machine learning.
MM: Is there a threshold, like X-amount of terabytes in which it becomes more effective?
RB: Absolutely. There are thresholds, but there’s not a general rule of thumb you can apply. It really depends on the kind of data that you have access to and the kind of prediction that you want to make. Whether you’re dealing with text data or image data or medical records data or click data, those all contain very different amounts of information, and it becomes a difference between making some kind of judgment about whether a person has found some resource or ad useful or intriguing versus trying to find some fine-grained demographic data about a person like what age were they, what gender were they, etc. As you ask more and more deep questions, you need more data.
MM: Machine learning has been around for a while. Why is it getting so much hype now?
RB: The main reason is machine learning systems have gotten way better over the last few years. Going from yesterday’s really terrible telephone number recognition – speaking into a telephone and having a machine barely understand you – all the way to basically being able to drive a driverless car around. Really the reason these methods have gotten better is three things: Folks have come up with new kinds of algorithms, but, even more importantly, computers have become so much more powerful that we can crunch on the third element, which is that we have so much data available now. There’s so much data now and so much computing power that algorithms that would have been impossible to apply five years ago are now commonplace.
TK: On the one hand, the amount of data available is very different from even 10 or 15 years ago. The other thing is that processing power got much more advanced, so you can do more things were previously possible. Plus, there’s a huge advancement in the techniques used. But the biggest influence is the tools available – tools people can use in an open source way. The whole tool ecosystem exploded. More people are using them.
MM: Do you think at some point AI will be able to make creative decisions in advertising?
TK: We are still far from that. Maybe eventually it might happen, but it’s hard to tell.
RB: Well, machine learning is already being applied to advertising, big time. Any of the ads that are placed in a Google search page, any of that is done via machine learning. But [regarding creativity], this is where we start to move from machine learning to artificial intelligence, which is a label that’s used for any problem that’s too hard to solve right now. I would say, currently, machine learning systems are extraordinarily effective in taking some training data – some data that they used to apply rules from – and then applying that data to new data. But really, they’re just learning some rules and applying them to a piece of data. Figuring out the creative process and how to mimic that in a machine is still an unsolved, very difficult problem.