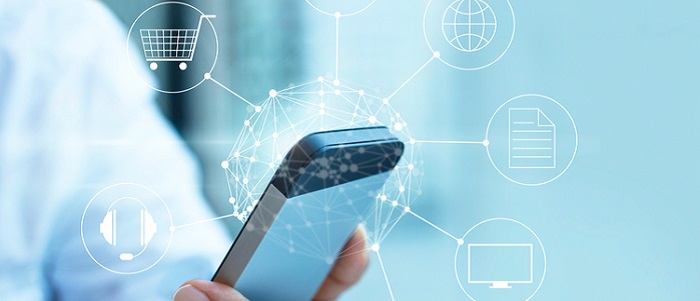
In this 4-part series, we continue to examine the four fatal flaws of attribution in today’s digital marketing landscape. Last time we discussed the second fatal flaw of Reliance on the Cookie and now tackle the third fatal flaw:
Fatal Flaw #3: Missing the Offline View
The Problem. Most digital marketing focuses on attributing online actions resulting from online media exposure. However, the majority of consumer purchases still occur offline. Integrating offline data—including customer profile data, modeled scores, response to offline campaigns, offline purchase data, and more—is important to online marketing efforts for at least three reasons. First is targeting: offline audiences that constitute attractive targets in the offline world (e.g., offline shoppers, modeled high-response consumers, etc.) are typically also attractive online, so marketers need the ability to on-board that data to maximize targeting scale. Second is measurement: the impact of digital advertising can extend to the non-digital world, so it’s critical that marketers understand when online media leads to offline impact, for accurate attribution. Third is optimization: an understanding of offline impact should drive smarter decisions about what digital media to buy, feeding into the machine learning that powers each digital decision. In addition, benefits also flow the other way, with online data able to inform paid and owned marketing in offline channels, enrich offline modeling and analytics (including Market Mix Modeling for deeper attribution insights), and more.
The Complication. Many marketers use offline-to-online match partners, such as LiveRamp, DataLogix, Neustar, and others to make offline data actionable in digital environments. However, the “match rates” (i.e., the percent of offline records matched to an online ID in a buying system such as a DSP) can often be in the 20-40 percent range. There are a variety of factors that can contribute to low match rates. Many matching systems rely on aforementioned cookie-based methods that cannot match audiences in cookieless contexts or across devices. Some fraction of the match data itself will always be inaccurate and out of date (as people move, create new logins, etc.). Even where a match is possible, falloff can occur simply because the online footprints of the match partner and the actual media execution system only partially overlap and consumers not seen by both systems can’t be matched. On top of it all, different match vendors generally employ different methods, like individual vs. household matching, deterministic vs. probabilistic matching, different match lookback windows, etc. The bottom line is that the majority of offline data points often go unmatched, leaving tremendous unrealized potential.
The Resolution. While 100 percent match rates will likely remain elusive, it’s clear there is room for improvement. Pushing offline to online match rates reliably into the 60-80 percent regime, or higher, would represent a dramatic increase in the power of offline data for targeting, attribution, and optimization. Achieving higher match rates starts with understanding that not all match partners are the same, and choosing the right match partner (or possibly multiple partners) suited to the type of CRM data in question. Better matching also requires best practices around data hygiene, including ongoing data cleansing and regular refresh of the data inputs. Importantly, the match process should not merely involve a mapping of cookies, but rather be based on a more robust solution that is actionable in cookieless web contexts, mobile in-app contexts, and across devices. It is also important to recognize that the match to an online ID is only the first step. What ultimately matters isn’t just how many consumers you match, but what fraction of those matched consumers you can actually target online. That means marketers must develop a complete understanding of the “match funnel” from an offline population, to the percent of those matched online, to the percent of those targetable within each media channel, the percent of those bid on, the percent of those won, and the percent of those ultimately attributable. Success requires understanding the dropoff at each stage to ensure the solution is strong across the entire funnel. The key is to work with a media execution partner that has the broadest actionable media scale across buying mechanisms, channels, and publishers to maximize fraction of matched consumers actually encountered in the wild.
Next time we’ll discuss the fourth and final fatal flaw: Failing to Measure Incremental Impact.