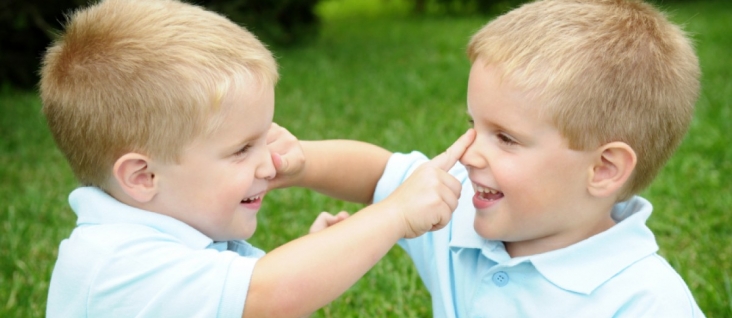
Shopping doesn’t end with the holidays, and neither does competition in the retail space. To keep their numbers up, retailers have to keep shoppers flowing into the store (online or otherwise). We recently spotlighted remarketing as a successful tactic to achieve this goal. This time, let’s talk about lookalike modeling and contextual targeting. Lookalike modeling can be used very effectively to prospect and drive new shoppers into the funnel. Contextual targeting refers to aligning advertising with content to increase the relevance of the advertising and ideally, advertising performance.
Lookalikes
Lookalike modeling is one of the best ways to put “big data” to work and drive new customer acquisition. Pulling data from first party data sets – POS, transaction, loyalty data or online behaviors – and combining that with other data on these consumers can create a detailed picture of who your best customers are across categories. With this information, marketers can create detailed profiles of each type of shopper. These profiles may include attributes like shopping behavior, product affinities, price sensitivities, devices used for shopping, and more. These profiles can then be used to target audiences with similar attributes – audiences that “look like” and “behave like” your best customers.
It’s important to note that the term “look-alike” often means different things to different people. On one end of the spectrum it can mean running a simple overlap report where you examine the packaged 3rd party data segments that over-Index the highest against your online “converters” or your best customers via your offline data set. A true look-alike modeled segment uses predictive algorithms to identify the unique attributes of a consumer group (pre-packaged targeting segments might include dozens of attributes). And to add even more complexity, the MediaMath “brain” – our decisioning algorithm – is really a model, determining the unique media characteristics and scenarios that are more predictive of your desired outcome. Let’s take a look at each of these options.
- Segment Overlap Reports
When a marketer is trying to reach a specific, yet finite audience, segment overlap reports can offer a quick, potentially cost-effective way to expand reach. These reports examine any online audience – an online converter (that might be aligned to a lower-funnel marketing tactic) or a homepage visitor (which might align more appropriately with a prospecting/upper-funnel tactic). In either case, marketers may want to find more people “like” that in an effort to acquire incremental customers. All platforms offer some sort of “Overlap report” that leverage pre-packaged segments from 3rd party data providers like eXelate, BlueKai, etc.. The downside to this option is that it is not a very precise method by which to identify unique audience attributes – the segments are a pre-packaged group of multiple attributes so it is absolutely a “broad stroke” approach. That said, it is easy to implement and depending on what the overlap analysis says, it can be very cost effective. For example, if the overlap analysis shows that your best customers are Moms with young children who enjoy NFL football, you can easily add contextual targeting to a buy or simply focus in on specific sites that reflect this audience or create whitelists that align with these audiences. Buying the 3rd party data segments themselves is also an option, but might be cost prohibitive based on the campaign goal.
Upside: can be cost effective & easily to implement
Downside: less precise
- Modeled Segments
Marketers can also leverage data companies like eXelate or AddThis to create true look-alike models that use complex predictive algorithms to identify very granular attributes & combinations of attributes that uniquely identify an audience the marketer is trying to replicate at scale. These companies have a tremendous amount of data that they piece together into targeting segments. But they can also use the individual data components that make up a segment to create modeled audiences that are more precise and therefore (hopefully) drive stronger performance. This option can also help marketers better understand their best customers and how they differ from other targets, hence making the entire media planning and targeting process smarter for current & future campaigns. In some cases, the biggest downside to this option is cost – depending on the campaign goal, adding a $1-2 data CPM on top of media costs can be prohibitive. But if the performance is high enough, the incremental cost is neutralized.
Upside: more precise
Downside: often require a minimum spend commitment which means optimizing away from this tactic if it’s not working isn’t an option
- MediaMath “Brain”
The “brain” is MediaMath’s machine-based decision algorithm that decides when to bid on an impression opportunity and what value to associate with that impression. It ingests a marketers campaign goal (sales, enrollments, downloads, application completes, etc.) and identifies the unique media characteristics that tend to result in that “conversion”. It then makes future bids decisions based on that information and continues to learn overtime as more conversions come in. While it’s not a look-alike product, the MediaMath brain is a predictive model that examines 20+ variables at once to optimizes a campaign run through the MediaMath platform in an automated fashion.
Upside: easy to implement, always on option to optimize campaigns, no incremental cost
Downside: NO DOWNSIDE!
Marketers looking to identify incremental consumers in a manner that is smarter than the “spray and pray” approach can leverage “look-alikes” via a programmatic marketing operating system like MediaMath’s T1 to reach these consumers at scale.
Contextual Targeting – A Perfect Compliment
Contextual targeting can lead to better campaign results as ads are viewed as less intrusive given their high relevance to adjacent content. This targeting tactic is also cost effective which also lends to success. A classic and simple example would be showing a shopper yoga pants as she’s reading an article about perfecting her warrior pose. She’s already thinking about yoga, so she’s likely to at least be interested in the yoga pants. Similarly, if a consumer is reading about the latest innovations in audio technology, new headphones might be really appealing. Contextual targeting is not “intent-targeting” – these folks have not necessarily expressed their intent to buy a product via a search or product site visit – but it can represent an opportunity to drive better performance as you reach consumers while they’re in the right “mindset”. Note that the best contextual tools will ensure that they’re relevant in a good way – so the ad for headphones doesn’t appear adjacent to content about the dangers of listening to music at high volumes.
Another highly valuable use of Contextual targeting is to define an advertiser’s brand safety policy – to some advertisers, celebrity gossip isn’t brand safe, but to others this is the perfect environment. Other options available via Contextual targeting include: showing up on pages with only 1-3 ad spots, high-visibility units, and those sites less likely to attract fraud.
Contextual targeting and lookalike modeling offer retailers a valuable method to reach shoppers in a smarter way – while they’re consuming relevant content or because they possess similar audience or media attributes as a group of consumers that have already proved themselves valuable to the retailer.
Ultimately, the beauty of the MediaMath platform is that you can test multiple targeting tactics like these to see what works best for your brand and then quickly and easily shift budgets toward the strategies that are working best.
To learn more about MediaMath’s solution, click here, and get more details about our retail capability here.